How ChatGPT is going to change the future of work and our approach to education — from livemint.com
From DSC:
I thought that the article made a good point when it asserted:
The pace of technological advancement is booming aggressively and conversations around ChatGPT snatching away jobs are becoming more and more frequent. The future of work is definitely going to change and that makes it clear that the approach toward education is also demanding a big shift.
…
A report from Dell suggests that 85% of jobs that will be around in 2030 do not exist yet. The fact becomes important as it showcases that the jobs are not going to vanish, they will just change and most of the jobs by 2030 will be new.
The Future of Human Agency — from pewresearch.org by Janna Anderson and Lee Rainie
Excerpt:
Thus the question: What is the future of human agency? Pew Research Center and Elon University’s Imagining the Internet Center asked experts to share their insights on this; 540 technology innovators, developers, business and policy leaders, researchers, academics and activists responded. Specifically, they were asked:
By 2035, will smart machines, bots and systems powered by artificial intelligence be designed to allow humans to easily be in control of most tech-aided decision-making that is relevant to their lives?
The results of this nonscientific canvassing:
-
- 56% of these experts agreed with the statement that by 2035 smart machines, bots and systems will not be designed to allow humans to easily be in control of most tech-aided decision-making.
- 44% said they agreed with the statement that by 2035 smart machines, bots and systems will be designed to allow humans to easily be in control of most tech-aided decision-making.
What are the things humans really want agency over? When will they be comfortable turning to AI to help them make decisions? And under what circumstances will they be willing to outsource decisions altogether to digital systems?
The next big threat to AI might already be lurking on the web — from zdnet.com by Danny Palmer; via Sam DeBrule
Artificial intelligence experts warn attacks against datasets used to train machine-learning tools are worryingly cheap and could have major consequences.
Excerpts:
Data poisoning occurs when attackers tamper with the training data used to create deep-learning models. This action means it’s possible to affect the decisions that the AI makes in a way that is hard to track.
By secretly altering the source information used to train machine-learning algorithms, data-poisoning attacks have the potential to be extremely powerful because the AI will be learning from incorrect data and could make ‘wrong’ decisions that have significant consequences.
Why AI Won’t Cause Unemployment — from pmarca.substack.com by Marc Andreessen
Excerpt:
Normally I would make the standard arguments against technologically-driven unemployment — see good summaries by Henry Hazlitt (chapter 7) and Frédéric Bastiat (his metaphor directly relevant to AI). And I will come back and make those arguments soon. But I don’t even think the standand arguments are needed, since another problem will block the progress of AI across most of the economy first.
Which is: AI is already illegal for most of the economy, and will be for virtually all of the economy.
How do I know that? Because technology is already illegal in most of the economy, and that is becoming steadily more true over time.
How do I know that? Because:
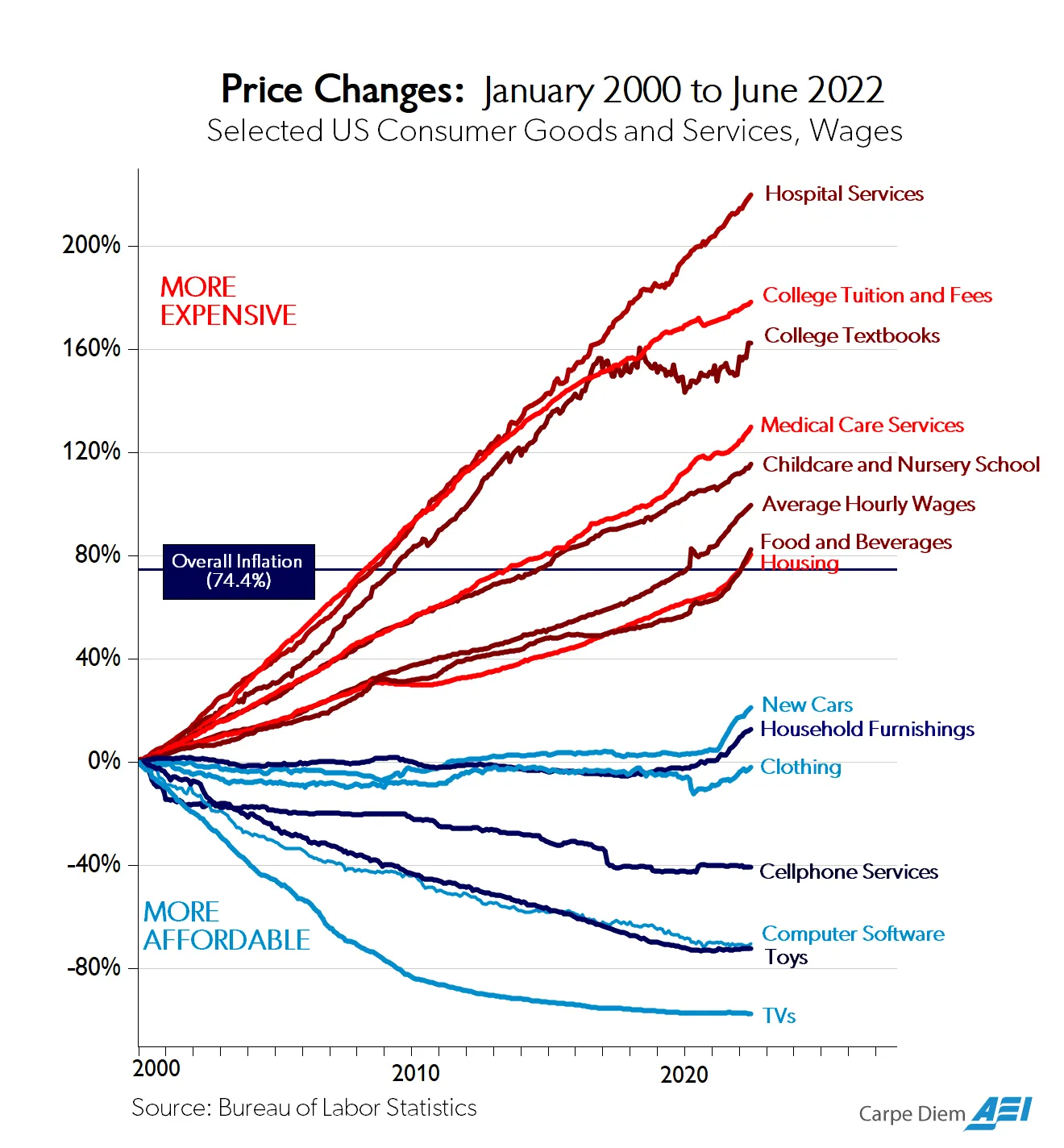
From DSC:
And for me, it boils down to an inconvenient truth: What’s the state of our hearts and minds?
AI, ChatGPT, Large Language Models (LLMs), and the like are tools. How we use such tools varies upon what’s going on in our hearts and minds. A fork can be used to eat food. It can also be used as a weapon. I don’t mean to be so blunt, but I can’t think of another way to say it right now.
- Do we care about one another…really?
- Has capitalism gone astray?
- Have our hearts, our thinking, and/or our mindsets gone astray?
- Do the products we create help or hurt others? It seems like too many times our perspective is, “We will sell whatever they will buy, regardless of its impact on others — as long as it makes us money and gives us the standard of living that we want.” Perhaps we could poll some former executives from Philip Morris on this topic.
- Or we will develop this new technology because we can develop this new technology. Who gives a rat’s tail about the ramifications of it?